Volume 28, Number 4—April 2022
Research
Genomic Epidemiology of Early SARS-CoV-2 Transmission Dynamics, Gujarat, India
Figure 2
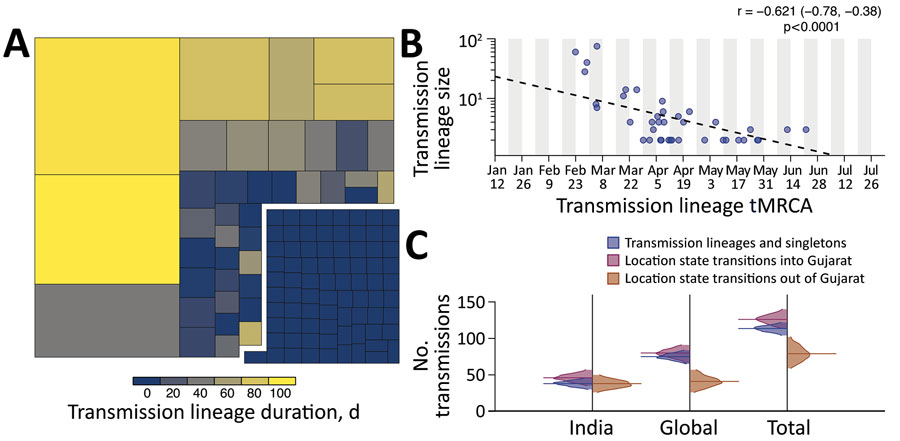
Figure 2. Size, duration, and importation of severe acute respiratory syndrome coronavirus 2 transmission lineages, Gujarat, India. A) Tree map summarizing the 113 detected transmission lineages by size. Colors indicate the duration of persistence of the lineage, and areas indicate the size of the transmission lineages. Lineage duration corresponds to time between the lineage’s oldest and most recently sampled genomes. B) Strong log‒linear relationship between size and mean tMRCA of each transmission lineages. Gray shading indicates time of testing; dashed line indicates slope. C) Breakdown of virus importations into Gujarat from other states in India or other countries. The number of location state transitions were estimated by using a robust counting approach (21) and a 3-location discrete trait phylogeographic analysis. tMRCA, time to most recent common ancestor.
References
- du Plessis L, McCrone JT, Zarebski AE, Hill V, Ruis C, Gutierrez B, et al.; COVID-19 Genomics UK (COG-UK) Consortium. Establishment and lineage dynamics of the SARS-CoV-2 epidemic in the UK. Science. 2021;371:708–12. DOIPubMedGoogle Scholar
- López MG, Chiner-Oms Á, García de Viedma D, Ruiz-Rodriguez P, Bracho MA, Cancino-Muñoz I, et al.; SeqCOVID-Spain consortium. The first wave of the COVID-19 epidemic in Spain was associated with early introductions and fast spread of a dominating genetic variant. Nat Genet. 2021;53:1405–14. DOIPubMedGoogle Scholar
- Murall CL, Fournier E, Galvez JH, N’Guessan A, Reiling SJ, Quirion P-O, et al. A small number of early introductions seeded widespread transmission of SARS-CoV-2 in Québec, Canada. Genome Med. 2021;13:169. DOIPubMedGoogle Scholar
- Lemieux JE, Siddle KJ, Shaw BM, Loreth C, Schaffner SF, Gladden-Young A, et al. Phylogenetic analysis of SARS-CoV-2 in Boston highlights the impact of superspreading events. Science. 2021;371:
eabe3261 . DOIPubMedGoogle Scholar - Komissarov AB, Safina KR, Garushyants SK, Fadeev AV, Sergeeva MV, Ivanova AA, et al. Genomic epidemiology of the early stages of the SARS-CoV-2 outbreak in Russia. Nat Commun. 2021;12:649. DOIPubMedGoogle Scholar
- Tegally H, Wilkinson E, Lessells RJ, Giandhari J, Pillay S, Msomi N, et al. Sixteen novel lineages of SARS-CoV-2 in South Africa. Nat Med. 2021;27:440–6. DOIPubMedGoogle Scholar
- Kraemer MUG, Hill V, Ruis C, Dellicour S, Bajaj S, McCrone JT, et al.; COVID-19 Genomics UK (COG-UK) Consortium. Spatiotemporal invasion dynamics of SARS-CoV-2 lineage B.1.1.7 emergence. Science. 2021;373:889–95. DOIPubMedGoogle Scholar
- Tegally H, Wilkinson E, Giovanetti M, Iranzadeh A, Fonseca V, Giandhari J, et al. Detection of a SARS-CoV-2 variant of concern in South Africa. Nature. 2021;592:438–43. DOIPubMedGoogle Scholar
- Volz E, Mishra S, Chand M, Barrett JC, Johnson R, Geidelberg L, et al.; COVID-19 Genomics UK (COG-UK) consortium. Assessing transmissibility of SARS-CoV-2 lineage B.1.1.7 in England. Nature. 2021;593:266–9. DOIPubMedGoogle Scholar
- Pandya RR, Raol MR, Mehta RA, Panchal JB, Chavda BK, Bhadarka KP, et al. Provisional population statistics as per 33 districts of Gujarat. Gujarat. Government of India; 2016 [cited 2022 Feb 24]. http://14.139.60.153/bitstream/123456789/13104/1/Statistical%20outline%20Gujarat%20state%202016.pdf
- Airport Authority of India. Traffic news for the month of March, 2020. p. 3 ‒5 [cited 2022 Feb 1]. https://www.aai.aero/en/business-opportunities/aai-traffice-news
- Ghosh RK, Tank N, Dutta M, Saxena S, Suthar P. Management of the COVID-19 pandemic in Gujarat: understanding the governance initiatives, leadership processes and their impact. Indian Institute of Management, Ahmedabad. 2020 [cited 2022 Feb 4]. https://www.iima.ac.in/c/document_library/Gujarat%20Covid%20Response%20Report-2020.pdf
- Joshi M, Puvar A, Kumar D, Ansari A, Pandya M, Raval J, et al. Genomic variations in SARS-CoV-2 genomes from Gujarat: underlying role of variants in disease epidemiology. Front Genet. 2021;12:
586569 . DOIPubMedGoogle Scholar - Schmieder R, Edwards R. Quality control and preprocessing of metagenomic datasets. Bioinformatics. 2011;27:863–4. DOIPubMedGoogle Scholar
- Parag KV. Improved estimation of time-varying reproduction numbers at low case incidence and between epidemic waves. PLOS Comput Biol. 2021;17:
e1009347 . DOIPubMedGoogle Scholar - Ferguson NM, Laydon D, Nedjati-Gilani G, Imai N, Ainslie K, Baguelin M, et al. Impact of non-pharmaceutical interventions (NPIs) to reduce COVID-19 mortality and healthcare demand. Imperial College London. 2020 [cited 2022 Feb 1]. https://www.imperial.ac.uk/mrc-global-infectious-disease-analysis/covid-19/report-9-impact-of-npis-on-covid-19/
- Gill MS, Lemey P, Faria NR, Rambaut A, Shapiro B, Suchard MA. Improving Bayesian population dynamics inference: a coalescent-based model for multiple loci. Mol Biol Evol. 2013;30:713–24. DOIPubMedGoogle Scholar
- Suchard MA, Lemey P, Baele G, Ayres DL, Drummond AJ, Rambaut A. Bayesian phylogenetic and phylodynamic data integration using BEAST 1.10. Virus Evol. 2018;4:
vey016 . DOIPubMedGoogle Scholar - Shapiro B, Rambaut A, Drummond AJ. Choosing appropriate substitution models for the phylogenetic analysis of protein-coding sequences. Mol Biol Evol. 2006;23:7–9. DOIPubMedGoogle Scholar
- Lemey P, Rambaut A, Bedford T, Faria N, Bielejec F, Baele G, et al. Unifying viral genetics and human transportation data to predict the global transmission dynamics of human influenza H3N2. PLoS Pathog. 2014;10:
e1003932 . DOIPubMedGoogle Scholar - Lemey P, Minin VN, Bielejec F, Kosakovsky Pond SL, Suchard MA. A counting renaissance: combining stochastic mapping and empirical Bayes to quickly detect amino acid sites under positive selection. Bioinformatics. 2012;28:3248–56. DOIPubMedGoogle Scholar
- Della Rossa F, Salzano D, Di Meglio A, De Lellis F, Coraggio M, Calabrese C, et al. A network model of Italy shows that intermittent regional strategies can alleviate the COVID-19 epidemic. Nat Commun. 2020;11:5106. DOIPubMedGoogle Scholar
- Balcan D, Colizza V, Gonçalves B, Hu H, Ramasco JJ, Vespignani A. Multiscale mobility networks and the spatial spreading of infectious diseases. Proc Natl Acad Sci U S A. 2009;106:21484–9. DOIPubMedGoogle Scholar
- Rader B, Scarpino SV, Nande A, Hill AL, Adlam B, Reiner RC, et al. Crowding and the shape of COVID-19 epidemics. Nat Med. 2020;26:1829–34. DOIPubMedGoogle Scholar
- Viboud C, Bjørnstad ON, Smith DL, Simonsen L, Miller MA, Grenfell BT. Synchrony, waves, and spatial hierarchies in the spread of influenza. Science. 2006;312:447–51. DOIPubMedGoogle Scholar
- Dalziel BD, Kissler S, Gog JR, Viboud C, Bjørnstad ON, Metcalf CJE, et al. Urbanization and humidity shape the intensity of influenza epidemics in U.S. cities. Science. 2018;362:75–9. DOIPubMedGoogle Scholar
- Zachreson C, Fair KM, Cliff OM, Harding N, Piraveenan M, Prokopenko M. Urbanization affects peak timing, prevalence, and bimodality of influenza pandemics in Australia: Results of a census-calibrated model. Sci Adv. 2018;4:
eaau5294 . DOIPubMedGoogle Scholar - Lu J, du Plessis L, Liu Z, Hill V, Kang M, Lin H, et al. Genomic Epidemiology of SARS-CoV-2 in Guangdong Province, China. Cell. 2020;181:997–1003.e9. DOIPubMedGoogle Scholar
1These senior authors contributed equally to this article.